From A to Ziti: Finding Hidden Meaning and Intent in Large Datasets
January 22, 2020
|
By:
Investigators experienced in interrogating data know that there may be more to a communication than meets the eye. Whether from intentional or unconscious behavior, clues abound.
When key facts are conveyed in nuanced or disguised language, it is important to explore the available collection of documents and communications with a linguistic and forensic sensibility. Although a difficult endeavor, the payoff is high when previously hidden meaning and intent surfaces in your investigation. In the process, individual finds often lead to a larger set of findings providing you a deeper understanding of who exactly knew or did what, and how they felt about it.
Follow the Scent
Unlike for a typical discovery request, searching for hidden meaning and intent in large data sets requires an ability to pinpoint nuanced — and often indirect — textual cues. This capability is distinct from relevance-based classification techniques such as TAR, which are optimized to ensure consistent coverage for a topic across a large data set. When looking for possible subterfuge or heightened emotion, for example, the task is more akin to incremental detective work than it is batch or prioritized classification. As such, it is useful to frame your efforts within an iterative search workflow that brings you into contact with potentially interesting content and communications, while also allowing you an ability to pivot off your search to explore particular key people, events, and timelines where interesting content appears to be clustered.
Make a List
An important first step in searching for key content you might otherwise be missing is to develop or tailor pre-existing lists of keywords and phrases targeting the types of behaviors and sentiments you are interested in uncovering.
For example, if you are investigating possible fraud, you may want to focus part of your search on isolating communications in which there are textual traces suggesting concealment. Some concealment-related phrases to add to a keyword list for a fraud investigation could include “do not share this,” “take off line,” or “delete email,” to name just a few.
Additionally, if you are interested in isolating internal chatter conveying strong concern or worry, you could include items like “atrocious,” “huge mistake,” “ill advised,” or “ordeal.”
Ziti? Or Fraud?
Apart from language expressing worry or concealment, other language worth targeting to get at hidden meaning and intent could include profanity and slang. Also, keep in mind the cultural context in which the communications and documents you are searching through were produced. For example, in a recent bribery and corruption case in NY state involving NY state government officials and private business executives, “ziti” (or “zitti”) was used as a code word to refer to bribes and extortion money. This particular code word in this context was borrowed from the language used by organized crime in New York and surrounding states.
Stay on Topic
Given the richness of language and culture, keyword lists targeting hidden figurative meaning can grow to hundreds, even thousands, of words and phrases. To avoid a deluge of hits, it is useful to pair these special keywords with broad issue indicators to make sure you are targeting not only figurative language, but also potentially relevant content. For example, if you are interested in isolating potential fraud around billing practices, one possible tactic would be to leverage proximity search by pairing fraud-related terms like “unusual” with a broad topical keyword term “billing” (e.g., unusual /50 bill[s,ed,ing]). Using this tactic in a systematic way across targeted sentiments and topics will get you a richer result set to focus your in-depth review on.
Prepare Ahead of Time
As with any search effort, setting up your data by threading email conversations and identifying near-duplicate sets of documents are two of the many approaches available to winnow down and prioritize the set of documents you perform targeted searches on. Techniques such as name normalization can also be especially helpful when your aim is to understand who is communicating with whom on a consistent basis.
Keep Smiling

It is also useful to explore how best to tailor the indexing of your data for searching — for instance, emojis are often used in key relevant conversations, yet they are rarely indexed automatically for search in review platforms. From both a discovery and investigative perspective, this can be a big blind spot. Preliminary research on the topic shows an increase in the number of US cases referring to emoji as evidence increased from 33 in 2017 to 53 in 2018.
Searching for key content conveyed through nuanced language is a complex task that is substantively distinct from relevance and topic classification. With the right mindset, workflow, and tools, you will be able to structure and manage this effort in order to isolate key facts otherwise left hidden that are relevant to your case.
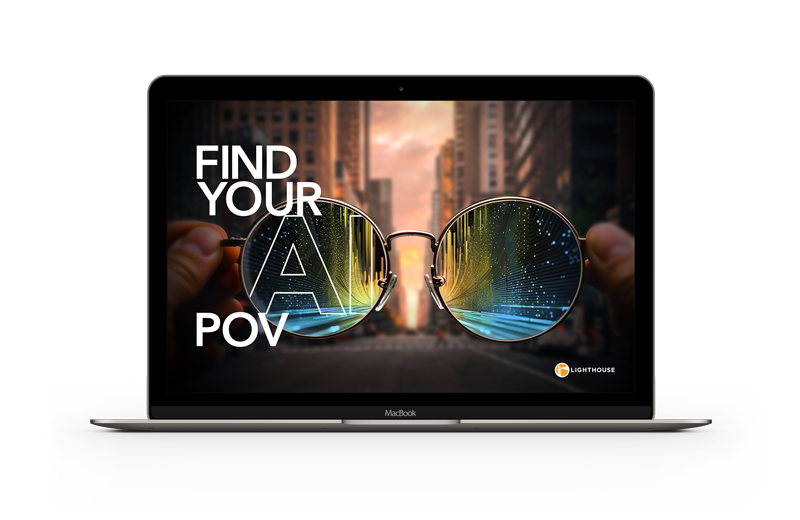