eDiscovery Analytics Use Cases You May Not Know About
March 23, 2021
|
By:
Evolving analytics tools and methods can help expedite review.
Analyze this! No, we’re not talking about the 1999 movie starring Robert DeNiro and Billy Crystal, but rather analytics mechanisms that many organizations are using today to streamline discovery. As these mechanisms become more sophisticated, it pays to keep abreast of the ways in which they can impact a review, including how data can be organized, visualized, identified and reduced.
For example, conceptual clustering can identify groups of topics that might be clearly responsive or non-responsive. Communication visualization maps can identify communication patterns of key parties within a data collection And, of course, predictive coding can train a supervised machine learning algorithm to identify potentially responsive and non-responsive documents based on classifications of other documents.
But there are other use cases for eDiscovery analytics many organizations aren’t taking advantage of that make eDiscovery workflows even more efficient and more cost effective. To improve the efficiency of eDiscovery workflows, organizations can now implement technology with the following analytics features.
Email Threading and Near Duplicate Identification
You may have heard the famous phrase “Insanity is doing the same thing over and over again expecting a different result.” But, in document review, insanity is simply doing the same thing over and over again. De-duplication using hash values identifies documents that are exact duplicates in content and format, but there is considerable additional content within document collections that is also duplicated within documents that aren’t exact matches. Email conversation threads contain considerable duplicative information, but conversations between multiple people can branch off, so you can’t just assume that the last message for the thread contains the entire thread discussion.
Documents converted to PDF may be identical in content but not format, so they have different hash values and are not “de-duped.” ESI collections often include multiple drafts of documents that have both duplicative and unique content. To avoid over-capture of duplicates and gain visibility into email branches, organizations can now employ advanced analytics that can help in the following ways:
- Utilize advanced algorithms to identify email thread relationships and individual emails in a thread with unique content
- Group similar documents with flexible near-duplicate identification to easily review and compare to determine whether the differences are significant
- Identify exact content duplicates with only formatting differences that hash de-duplication would not catch.
Name Normalization and Entity Analysis
What’s in a name? Potentially, a whole lot of options! If the sixth US president were alive today and sending emails, here are some ways that you might see him represented within the collection:
- John Adams
- Johnny Adams
- John Q. Adams
- Q. Adams
- Quincy Adams
- Adams, John
- Adams, John Q.
- Adams, J.Q.
- Adams, J. Quincy
- jadams@xyzcorp.com
- /O=XYZCORP/OU=EXCHANGE ADMINISTRATIVE GROUP (FYDIBOHF23SPDLT)/CN=RECIPIENTS/CN=jadams
- Adams@gmail.com
- And potentially more…
That’s a lot of variation – just for one person! Case teams often waste significant time and energy sorting through the numerous variations of names and email addresses for individuals in a matter. Advanced analytics solutions can be used to automated name normalization algorithms to link different name variations and email addresses to a single individual, format those names uniformly and aggregate the normalized participants that appear across an entire email thread group. The result? Refined results that streamline processes such as privilege logging without the intensive manual cleanup typically associated with the process.
Metadata Analytics
AI-driven analytics applied to the metadata can streamline eDiscovery by:
a) identifying mass email communications so that reviewers can focus on more likely responsive emails;
b) filtering email signature images and other extraneous embedded objects; and
c) remediating data populations with missing or incomplete metadata by auto-detecting and populating email metadata fields on inbound productions.
Privilege Analytics
Automated categorization and classification powered by advanced analytics can also be applied to privilege review to weed out non-responsive and non-privileged material early and rapidly identify, elevate and prioritize potentially privileged information. Customizable rules to exclude disclaimers and boilerplate language can also improve the accuracy of that identification process by eliminating many false positives.
As most privilege determinations involve considerations of nuance and context, human judgments are a necessary part of the process. Pre-built and customized linguistic models, name normalization and email thread identification can extend those automated privilege determinations more quickly through the collection, with automated identification of legal concepts, privilege actors and law firms and a reusable asset with consistent propagation of privilege designations across matters.
And clean name normalization outputs, along with automated and customizable privilege reasons assigned to each document expedite privilege log creation, significantly decreasing the manual cleanup often associated with this time-consuming task.
Personal Identifiable Information (PII) Detection
Finally, with all of the data privacy requirements associated with recent regulations such as the General Data Protection Regulation (GDPR) and the California Consumer Privacy Act (CCPA), identifying and protecting PII has become a requirement within every phase of the eDiscovery lifecycle. Using analytics and pattern matching through regular expressions (RegEx) to identify common format numbers such as passport IDs, social security numbers, drivers license numbers and credit card numbers, as well as identification of common form types that often contain PII (such as loan applications or IRS forms) will help flag those documents so that they can be adequately protected throughout the process.
Newer, more advanced AI-driven analytics solutions go a step further by utilizing highly precise classifiers to model the way in which different forms of supported personal data appear in data populations. These automated solutions provide rapid identification of likely and potential PII, resulting in rapid insights and immediate access to the most relevant documents first.
Conclusion
You may be using analytics to streamline parts of your eDiscovery process, but there are always new use cases being identified to leverage analytics to make your eDiscovery workflows more efficient. Even Analyze This had a sequel!
For more information on ways H5 Matter Analytics® can assist your organization in creating efficiencies and expediting eDiscovery workflows, click here.
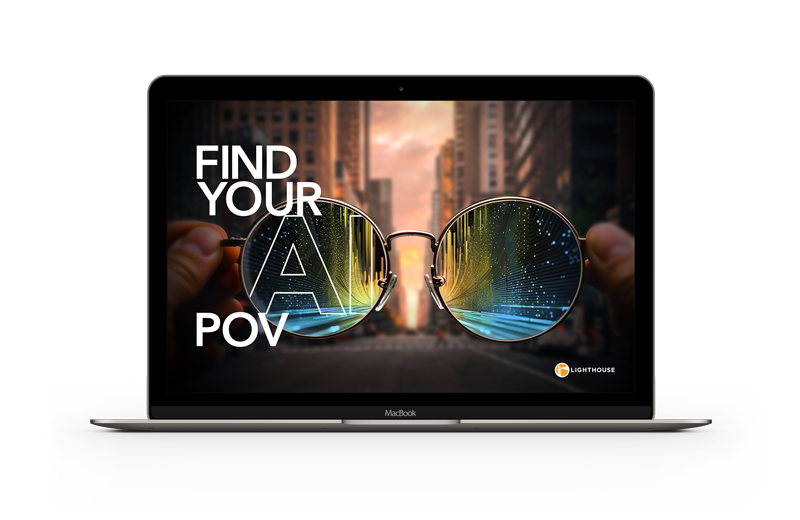